Welcome to the Abbasi Lab Homepage!
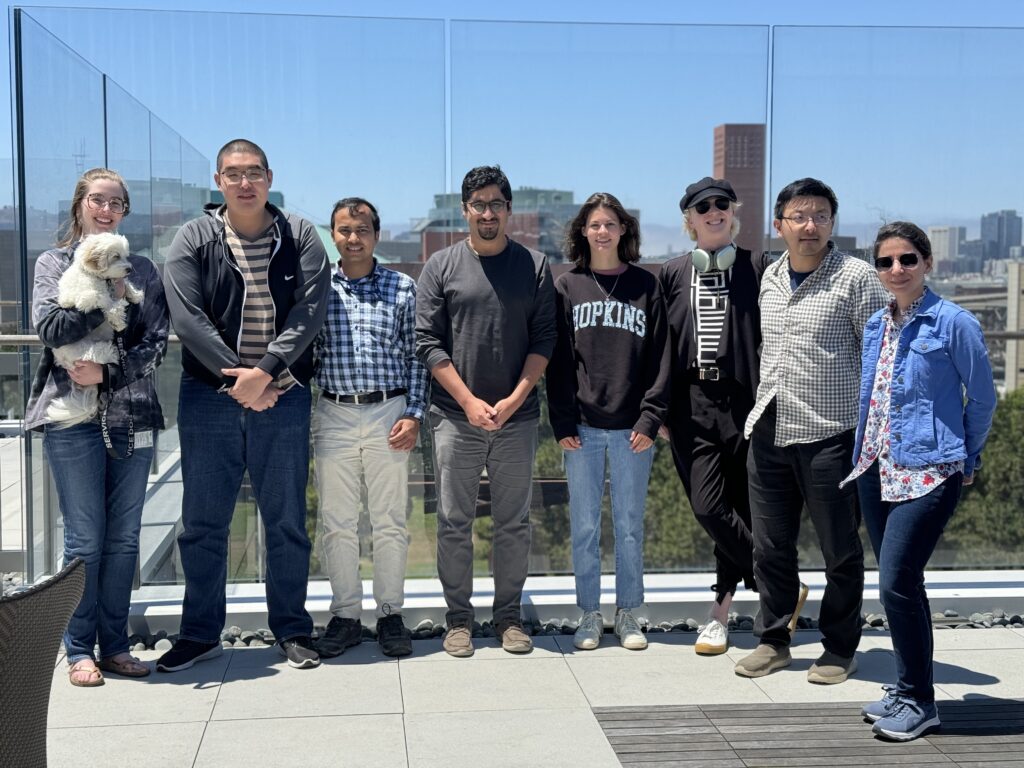
We are a research team at the University of California, San Francisco where we investigate the role of advanced computational tools in understanding brain functions and its related disorders. More specifically, our research program revolves around the development of interpretable machine learning tools to (1) integrate multi-modal data collected from the brain (and body) in both microscopic and macroscopic resolutions, (2) predict functions of biological systems in different resolutions, and (3) determine the functional differences across neurological disorders. We are a part of the Neuroscape Center and Cognitive Neuroscience division at UCSF and our team is supported through funding from NIH National Institute of Mental Health, NIH National Institute of Aging, Weill Neurohub, Sandler Program for Breakthrough in Biomedical Research, UCSF Innovation Ventures, and Google.
We are hiring!
We have multiple openings for postdoctoral fellows to work with us at UCSF on exciting applied problems at the intersection of machine learning and computational/clinical neuroscience. Please see Openings for more information or directly contact Reza via Reza.AbbasiAsl@ucsf.edu to learn more!
News and Events
July 2024: New paper accepted in PNAS: Unsupervised pattern discovery in spatial gene expression atlas reveals mouse brain regions beyond established ontology.
July 2024: UCSF News coverage of our recent paper: Breakthrough: How AI Uses Videos to Gauge Parkinson’s Severity.
July 2024: New software released: 7T MRI synthesization from 3T acquisitions.
July 2024: New perspective piece on arXiv: Opportunities in deep learning methods development for computational biology.
June 2024: New software released: Biomedical question answering robustness benchmark.
June 2024: Gavin’s paper is now accepted in MICCAI 2024: 7T MRI Synthesization from 3T Acquisitions.
June 2024: Congrats Alex for receiving the UCSF GRFM fellowship!
June 2024: Naomi joined the lab! Welcome Naomi!
June 2024: New paper out in the Journal of Neural Engineering: Structurally-constrained encoding framework using a multi-voxel reduced-rank latent model for human natural vision.
June 2024: Maria‘s paper is highlighted as Editor’s Choice in Brain: Motor network gamma oscillations in chronic home recordings predict dyskinesia in Parkinson’s disease.
May 2024: New paper accepted in npj Parkinson’s Disease: Interpretable Video-Based Tracking and Quantification of Parkinsonism Clinical Motor States.
May 2024: New paper accepted in GEN Biotechnolgy: Machine Learning for Uncovering Biological Insights in Spatial Transcriptomics Data.
May 2024: New preprint out: Data-driven fine-grained region discovery in the mouse brain with transformers.
May 2024: Reza presented an Invited Lecturer at the Caltech Bioengineering Lecture Series.
March 2024: New preprint out: 7T MRI Synthesization from 3T Acquisitions.
Feb 2024: New preprint out: Zero-shot sampling of adversarial entities in biomedical question answering.
Jan 2024: Congrats Arefeh for winning the second place prize in the Vesuvius challenge!
Jan 2024: Maryam joined the team! Welcome Maryam!
Jan 2024: Maria’s paper is published in Brain: Motor network gamma oscillations in chronic home recordings predict dyskinesia in Parkinson’s disease
Jan 2024: Reza is presenting an invited talk at ETH Zurich.
Dec 2023: Reza is organizing an invited session at CMStatistics 2023 on Interpretable Machine Learning for Scientific Discovery!
Nov 2023: New software released: https://github.com/abbasilab/Video-Tracking-PD.
Nov 2023: New preprint out: Interpretable Video-Based Tracking and Quantification of Parkinsonism Clinical Motor States.
Nov 2023: New preprint led by R. Carhart-Harris: The entropic heart: Tracking the psychedelic state via heart rate dynamics.
Nov 2023: Max joined the lab! Welcome Max!
Nov 2023: Alex is presenting his work at MLCB 2023.
Nov 2023: Kara is presenting her work at the SfN nanosymposium on Neural Decoding and Neuroprosthetics.
Sep 2023: Our book chapter on Interpretable Prototype Discovery in Deep Learning-based Time Series Classification accepted to be published by Springer.
Aug 2023: Reconstructing visual experience from a large-scale biologically realistic model of mouse primary visual cortex abstract now published in Journal of Vision.
Aug 2023: Congrats to Aelx for his recognition as BMI Discovery Fellow!
Aug 2023: Congrats to Alex for winning the UCSF ARCS Scholarship award!
July 2023: Abstracts from the lab accepted in ISMB 2023, OCNS 2023, and RECOMB-Seq 2023 on our models for spatial transcriptomics data.
July 2023: Reza is appointed as the Executive Committee Member for the Bakar Computational Health Sciences Institute.
June 2023: Reza presented lab’s recent work at the 9th Annual BRAIN Initiative Meeting.
June 2023: Kara presented her work at 10th Annual BCI meeting.
May 2023: Russell joined the lab! Welcome Russell!
April 2023: Diurnal Step Count Patterns in Progressive Multiple Sclerosis abstract now published in Neurology.
April 2023: Congrats to Alex for passing his qualifying exam!
April 2023: Congrats to Daniel for receiving 2023 URAP Summer Fellowship Award!
March 2023: Congrats to Alex for receiving the Honorable Mention on his NSF GRFP application!
March 2023: New preprint out: Machine Learning for Uncovering Biological Insights in Spatial Transcriptomics Data.
March 2023: New software released: https://github.com/abbasilab/osNMF
March 2023: New preprint on unsupervised pattern discovery in large-scale spatial gene expression datasets.
March 2023: New editorial published in Front. Comput. Neurosci.
March 2023: Kara joined the lab! Welcome Kara!
Feb 2023: Reza will be giving a talk at ITA 2023 in San Diego.
Feb 2023: Congrats to Rob for getting admitted into the UCSF BMI PhD program!
Feb 2023: One abstract accepted for presentation at AAN 2023.
Feb 2023: One accepted oral presentation at VSS 2023.
Jan 2023: We received the Kunal Patel Catalyst Award for our project on camera-based tracking of PD in collaboration with the UCSF Movement Disorders center!
Dec 2022: We are now a part of UC Berkeley/UCSF joint graduate program in Computational Precision Health. Consider applying if you want to join our lab! Read more here.
Dec 2022: Reza will be presenting an invited talk at CMStatistics 2022.
Dec 2022: Reza will be presenting as a panelist at the NIH Brain Initiative Workshop on High Throughput Imaging Characterization of Brain Cell Types & Connectivity.
Nov 2022: Jen joined the lab as our new research data analyst!
Nov 2022: Congrats to Gavin for passing his qual exam!
Nov 2022: Reza gave the UC San Diego NanoEngineering/Chemical Engineering seminar.
Nov 2022: We will be at SfN in San Diego. Reach out if you are around!
Nov 2022: Maria will be presenting a poster at SfN 2022 titled Neural spectral features capture severity of dyskinesia in Parkinson’s Disease.
Nov 2022: Thank you Google for funding our project on the applications of wearables in Parkinson’s disease!
Nov 2022: Reza is featured in the Neuroscape Network newsletter.
Aug 2022: Alex joined the lab as a new PhD student!
July 2022: We received the lab’s first NIH/NIMH R01 grant to investigate the role of ML in integrating structural and functional neural data!
July 2022: Reza will give a talk at CNS 2022 on the functional characterization of an entire column in mouse V1.
July 2022: Reza will present recent work on the compression of voxel-wise models at FENS 2022.
July 2022: Reza received the UCSF Neurology N-RIP award!
July 2022: New preprint on Compression-enabled interpretability of voxel-wise encoding models.
May 2022: Rob, Maria, and Zhinoos joined the lab!
May 2022: Reza will speak at the 24th International Conference on Computational Statistics in August.
April 2022: Reza will speak as the community speaker at the American Association of Neurological Surgeons Annual Meeting 2022.
March 2022: Two accepted oral presentations at Sharif Neuroscience Symposium.
Feb 2022: Reza will present as the plenary speaker at the ICRTB 2022.
Feb 2022: Our paper on the robust registration of MRIs is now published in Algorithms.
Jan 2022: We have been awarded a new multi-institution grant from Weill Neurohub to develop ML-based computational tools for the integrated analysis of 7T and 3T MRIs.
Jan 2022: We have received a second New Frontiers Research Award from the Sandler Program for Breakthrough Biomedical Research.
Dec 2021: Reza is presenting as an invited speaker at the CMStatistics 2021.
Oct 2021: Roozbeh Farhoodi joins the lab as a new postdoctoral scholar. Welcome, Roozbeh!
August 2021: Congrats to Austin Jang and Shiladitya Dutta, two undergraduate students in the lab, for winning the UC Berkeley URAP fellowship for their research projects!
August 2021: New paper published in Frontiers in Big Data: Structural Compression of Convolutional Neural Networks with Applications in Interpretability.
June 2021: New paper on arXiv: Multi-Modal Prototype Learning for Interpretable Multivariable Time Series Classification.
June 2021: Congrats to Austin Jang and Shiladitya Dutta, for winning the URAP summer fellowship!
May 2021: We got awarded the New Frontiers Research Award from the Sandler Program for Breakthrough Biomedical Research.
May 2021: We are hiring a fully-funded postdoc fellow to lead efforts for our new Neuroscape-based project on computational models of multi-modal biosensor data! Reach out if you are interested!
May 2021: Gavin Cui joins the lab as a bioengineering PhD student. Welcome Gavin!
April 2021: Our NIH grant in collaboration with Neuroscape PIs (Ted Zanto and David Ziegler) on “Neural markers of impending task performance” just got funded!
Feb 2021: One abstract accepted at VSS 2021 on our large-scale standardized survey of neural receptive fields in an entire column in mouse V1.
Feb 2021: We are hiring two new postdoc fellows to join us at UCSF and lead our efforts on developing a computational pipeline to analyze spatial gene expression in mice.
Feb 2021: Reza will be giving a talk at the faculty seminar at the Kavli Institute.
Jan 2021: Reza becomes a Weill Neurohub Investigator.
Jan 2021: We got awarded the Weill Neurohub Next Great Ideas Fund! Our proposed project is focused on spatial gene expression analysis in mice.
Nov 2020: Reza will give an invited talk at the 2020 Basic and Clinical Neuroscience.
Oct 2020: One abstract accepted at CSHL NAISys meeting on interpretable models of neurons in visual cortex.
August 2020: One abstract accepted at JSM 2020 on Revealing Spatial Gene Patterns and Interactions in Mouse Brain via Stability-Driven NMF.
May 2020: One abstract accepted at CNS 2020 on compressed models of neurons in V4!
May 2020: Our paper featured on the cover of Neuron!
March 2020: Reza is now a joint faculty at the Department of Bioengineering and Therapeutic Sciences at UCSF.
March 2020: We are now officially affiliated with Neuroscape!
March 2020: The dataset from our recent eNeuro paper is now available on the CRCNS data repository.
March 2020: Here is a nice read from Allen Institute on our recent Neuron paper.
March 2020: Due to the recent Corona Virus outbreak, the lab has moved to fully remote mode! Hope everyone is staying safe and healthy!
March 2020: We are happy to welcome to the lab Oluwaseun Adegbite as rotation PhD student, Luke Chen as an RA, and five UC Berkeley undergraduates through the URAP program: Kevin Lin, Rohan Divate, Austin Jang, Anmol Parande, Felix Ching!
Feb 2020: Reza will give an invited talk at ITA 2020 on interpretation of neural networks with applications in comp neuroscience.
Jan 2020: We will be present at Cosyne 2020 with one invited talk and one accepted abstract on compressed interpretable models of neurons. Reza will give the invited talk at the workshop on “Scrutinizing models of brain function: from in-silico stimulus synthesis to direct brain perturbation”.
Jan 2020: Our paper titled “Systematic Integration of Structural and Functional Data into Multi-Scale Models of Mouse Primary Visual Cortex” has been accepted at Neuron.
Jan 2020: Our paper titled “Superficial bound of the depth limit of 2-photon imaging in mouse brain” appeared on eNeuro.
Jan 2020: We are now affiliated with Bakar Computational Health Sciences Institute at UCSF! Reza will be a faculty affiliate at the institute.
Dec 2019: Welcome to the lab Neel! Welcome Neel!
Nov 2019: Welcome to the lab Ahyeon and Franklin!
Nov 2019: Reza joined the UC Berkeley-UCSF graduate program in bioengineering. We have multiple openings for rotation students!
Oct 2019: Our paper titled “Definitions, methods, and applications in interpretable machine learning” appeared on PNAS.
Oct 2019: Reza is presenting at SfN on functional imaging of excitatory neurons in an entire column in mouse visual cortex. Here is the abstract.
Sep 2019: Abbasi Lab officially started!